
At the forefront of surgical video analytics, SDSC is leveraging cutting-edge Machine Learning (ML) to transform the way surgeons analyze their work. Currently, SDSC has developed 11 tailored ML models for tool detection, tool classification, and surgical procedure breakdown, enabling meticulous post-operative review of a variety of surgical procedures. To better understand the effectiveness of these models, SDSC’s ML engineers conducted comprehensive performance evaluations using five distinct benchmark datasets.
This benchmarking process aimed to assess the effectiveness of SDSC models across various surgical domains. This included in-domain datasets, where models were tested on data similar to their training data, and cross-domain performance, where models were tested against entirely different surgical procedures. The results yielded 19 benchmark comparisons, offering crucial information about the models’ strengths and areas for improvement. These insights allow for a deeper understanding of the models’ training processes, performance metrics, and responses to cross-domain data variability.
The evaluated models covered multiple surgical procedures and were tested for surgical tool detection, tool classification, and phase classification. Benchmark datasets included: Cholec80 (laparoscopic cholecystectomy surgeries), CholecT50 (a subset of Cholec80), Endoscapes (laparoscopic cholecystectomy surgeries), SOCAL (Simulated Outcomes following Carotid Artery Laceration; cadaveric surgeries), and PitVis (endoscopic pituitary tumor surgeries). Each dataset was carefully matched with SDSC’s models, ensuring compatibility by aligning tool and procedural class ontologies. This allowed for successful performance evaluation while accounting for variations in tool appearances, annotations and procedures.
Benchmarking metrics used included: precision, recall, and mean average precision (mAP) score (mAP50 and mAP50-95). While precision and recall measures the accuracy of tool classification, mAP50-95 assesses the overlap between predicted and ground truth bounding boxes. The closer the value is to 1, the higher the overlap.
Endoscopic Endonasal Approach Tool Detection Benchmark
The Endoscopic Endonasal Approach tool detection model, trained on endoscopic endonasal surgery, performed exceptionally well on in-domain datasets like SOCAL. Interestingly, when applied to entirely different areas of the body like Cholec80 and CholecT50 (datasets for gallbladder surgery), the model still achieved notable success. Despite being trained on procedures involving the sinuses, skull, and brain, the model performed remarkably well in detecting common tools like the Grasper (see figure below) in abdominal surgery videos as well.
This unexpected result highlighted the potential for SDSC models to be cross applied beyond their original training domains, suggesting promising generalizability across diverse surgical procedures.
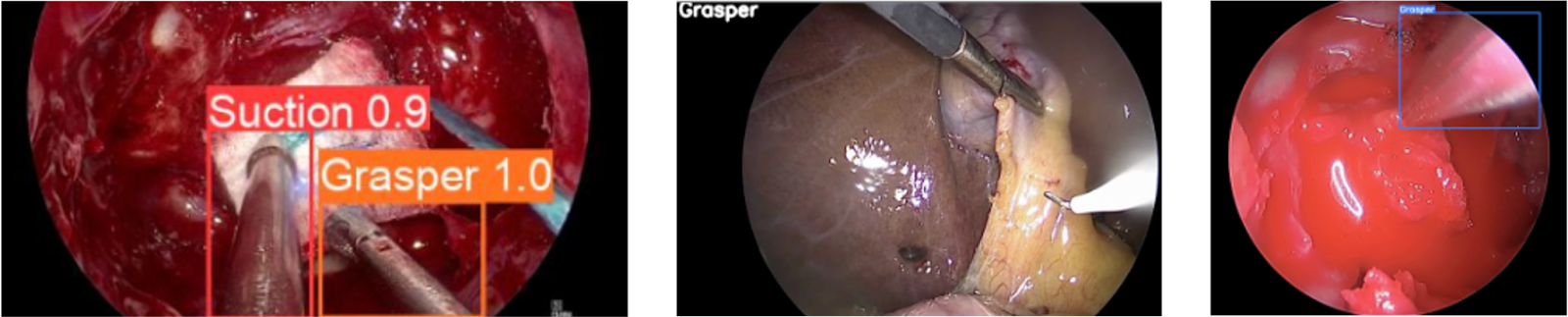
Laparoscopic Cholecystectomy Tool Detection Benchmark
For laparoscopic cholecystectomy (gallbladder removal surgery), SDSC models excelled on in-domain datasets like Cholec80, CholecT50 and Endoscapes. However, when applied to the SOCAL dataset, a cross-domain challenge occurred due to differences in annotation styles. Another important finding was the low result for the Endoscapes benchmark, despite this being an in-domain comparison. The Cholecystectomy model was trained only to detect tooltips, whereas the Endoscapes dataset ground truth labels were for whole tools. This resulted in a low intersection-over-union value, and subsequently a lower mAP score (See Figure Y). This variation in annotation emphasizes the importance of consistent labelling practices when comparing benchmark datasets.

Ectopic Pregnancy Surgery Simulation Tool Detection Benchmark
The Ectopic Pregnancy Surgery tool detection model presented a unique benchmarking case. Trained exclusively on practice surgical videos from ALL SAFE, rather than those from real patients’ surgeries, all benchmark datasets tested were considered cross-domain. Despite this, the model performed exceptionally well on its own validation data. However, a significant performance gap emerged when tested against the Endoscapes dataset, partly due to faulty ground truth annotations where overlapping bounding boxes were present.

Pituitary Tumor Surgery Procedure Breakdown Benchmark
The Pituitary Tumor Surgery procedure breakdown benchmark was designed to identify the phases of pituitary tumor surgery. While the SDSC model performed exceptionally on both its internal validation dataset and the PitVis dataset (phase classification dataset), a challenge arose in maintaining a fair evaluation. SDSC’s model had already seen some of the PitVis dataset during training, leading to potentially inflated results (see figure below). A truly unbiased evaluation would require training the model on the same validation split used by the PitVis dataset to ensure an accurate “apples-to-apples” comparison. SDSC will use this approach going forward, training models exclusively on benchmark validation splits to achieve a fair evaluation.
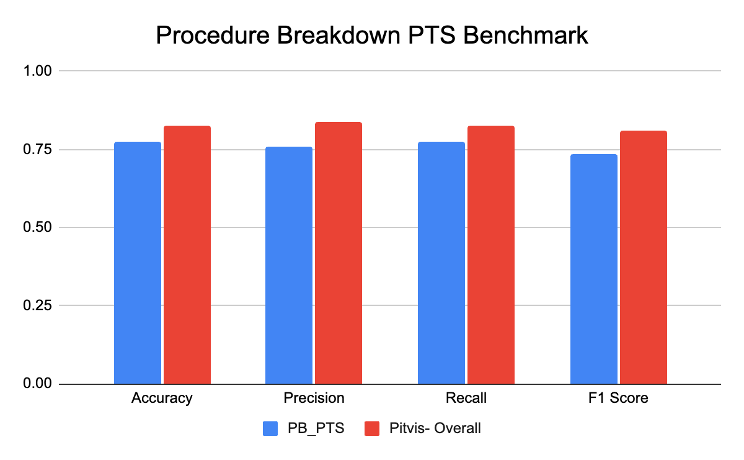
Challenges in Benchmarking Surgical Video Models
A significant methodological challenge in this benchmarking effort was class ontology mapping. SDSC’s models use a specific set of tool labels, while benchmark datasets may use different labels or characterize tools differently. For example, a suction tool might be labelled as “class 0” in SDSC models, but “class 2” in a benchmark dataset. Careful realignment of class indices and label mapping between datasets ensured valid and consistent model evaluation.
Moving forward, SDSC plans to refine its benchmarking practices, including standardizing validation splits and further explore annotation consistency across datasets. For example, datasets like Cholec80 don’t have bounding box locations, and therefore work only for tool classification, rather than detection. In cases like this, overlap between predicted and ground truth bounding boxes is not accounted for, so any tool detections are automatically classed as “1”. As such, mAP metrics were not directly applicable, and so an adjusted evaluation approach was required.
Pioneering the Future of Surgical Video Analytics
The most exciting finding from this benchmarking effort was the potential for cross-domain application of SDSC’s models. While current models are procedure-specific, their ability to perform reasonably well on entirely different surgical datasets opens the door to developing generalized models with a single robust architecture. With models capable of analyzing multiple surgical procedures, SDSC could significantly simplify deployment and improve scalability.
SDSC’s benchmarking efforts mark a critical step toward revolutionizing surgical video analysis. By evaluating our models against both in-domain and cross-domain datasets, we’ve gained a deeper understanding of their strengths and limitations.
This knowledge will guide the development of next-generation ML models that empower surgeons with actionable insights, improve surgical workflows and ultimately enhance patient outcomes.
We invite surgeons and surgical residents to collaborate with us in advancing surgical and clinical research. By leveraging our cutting-edge surgical video analytics, together we can drive innovation, improve patient outcomes, and shape the future of surgical care.